We hear a lot these days about “content targeting”, “customer profiling”, “customer segmentation” and “adaptive content” but with very little groundwork being done first to define who and what we mean by a Customer.
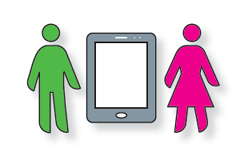
For the purposes of this article we will assume that Customer and User are the same thing, but the point is, they are not the same thing as a Person and not the same thing as a Device or Profile (cookie) either.
Here’s a few examples to illustrate what we mean by this.
If we have a Person (an individual human being) in a household that uses a PC to browse the web and enters some data while shopping, we might establish that they are 45 years old and Male. We might also infer that they like Golf and Cycling based on some content views or ad clicks.
But if we have a different Person in the same household using the same PC to browse the web (using the same profile), we might then find out that they like skin care products and ladies fashion. This could be a cross dressing male golfer and cycler with very good skin, but probably not. Its probably the wife or maybe a teenage daughter? But in our customer database we only have one record (probably associated to one cookie).
So the point here is that there is a one-to-many relationship between a web Customer definition, and a real Person. A Customer on the web might actually be a collection of multiple people.
Alternatively let’s say we have two children using an iPad at the same time to play a game on the web. Two Persons, one Customer record.
The reverse is also true. Jane might log in as jane@hotmail.com to book a holiday on Expedia but log in as jane.smith@company.com to book a business flight. That’s two Customer records, but one real Person. If we get the chance to link these two Customer records up to one Person (by phone number or some other common snippet of data), this might help our targetting. But it might make it a lot worse. Maybe Jane doesn’t want to see highly targeted ads or offers relating to her personal interests when she is busy booking a work trip, or maybe she has given her PA the login to do it or her behalf.
Then we bring in the Device dimension. If a Person has an iPhone and a laptop, that is two Customer records (assuming anonymous cookie identification, consolidated into one following login), but two Devices.
As you can see it gets pretty complex pretty quickly. The point is that if we are going to talk about “the Customer” or “personalising the Customer experience” or “targetting a Customer segment”, we had better define what we mean by a Customer first, as this is not as obvious as it sounds, and we had better also measure our confidence in what we think we know about that Customer really being true.
- Do we know for sure that our Customer record represents is a single individual Person?
- Do we know for sure that our Customer record represents at least two different Persons?
- Do we not even know yet what our understanding of the Customer and do we have indicators that the Customer record is almost certainly wrong or contradictory?
In statistics it is standard practice to calculate or measure the accuracy or certainty of the stats before we start using them.
So we need measures of how certain we are that someone is who we think they are. If we can use software and data analytics to calculate that certainty, then we can build targeting rules that only kick in if the certainty is above a threshold.
For example, mobile phones are closely associated with an individual person (they are rarely shared). So if we receive data from a mobile device indicating that the Customer is female, and a day later some data indicating that they like football, we have a fairly strong indicator that we have a female football fan, even though that might be a fairly small and low-probability segment, and we can start targeting them accordingly. If both pieces of data were collected during working hours, reducing the likelihood that a male Person lent their phone to a wife or girlfriend to access the web, the correlation of the two data points is even higher and gives us even greater certainty.
But if we had received the same two data points via an iPad, at different times of the day, it is extremely likely that we have two different users here, perhaps a wife and a football-fan husband who used the iPad at different times.
There was never a one-to-one mapping between Devices and individual Persons and this has been a limitation of personalisation on the web since the 90s. But today’s multitude of devices and increasing number of shared household devices, both makes the problem more tricky (the iPad example) and easier (the phone example) to work out who we are really dealing with when trying to target or personalise content.
On top of this we also have Profiles, allowing multiple Persons to have their own isolated data and identities on the same Device.
At this time there are very few technologies that help address this problem, which is why it is currently a key area of research for Priocept. But it’s vital to get the basics right and define what we mean by a Customer, a Person, a Device and a Profile, at the start of a personalisation, content targetting or analytics project, and understand the complex relationships between them. Without this it’s all too easy to draw completely nonsense conclusions from the most sophisticated profiling, targeting and analytics solutions that can be built. Garbage in, garbage out.
In addition to the significant challenges outlined above, the new EU legislation around use of cookies is sure to cause additional headaches for the pioneers in this arena, especially those offering 3rd party cross-site profiling. It will be interesting to see how technology develops and evolves to solve these problems over the coming months and years.